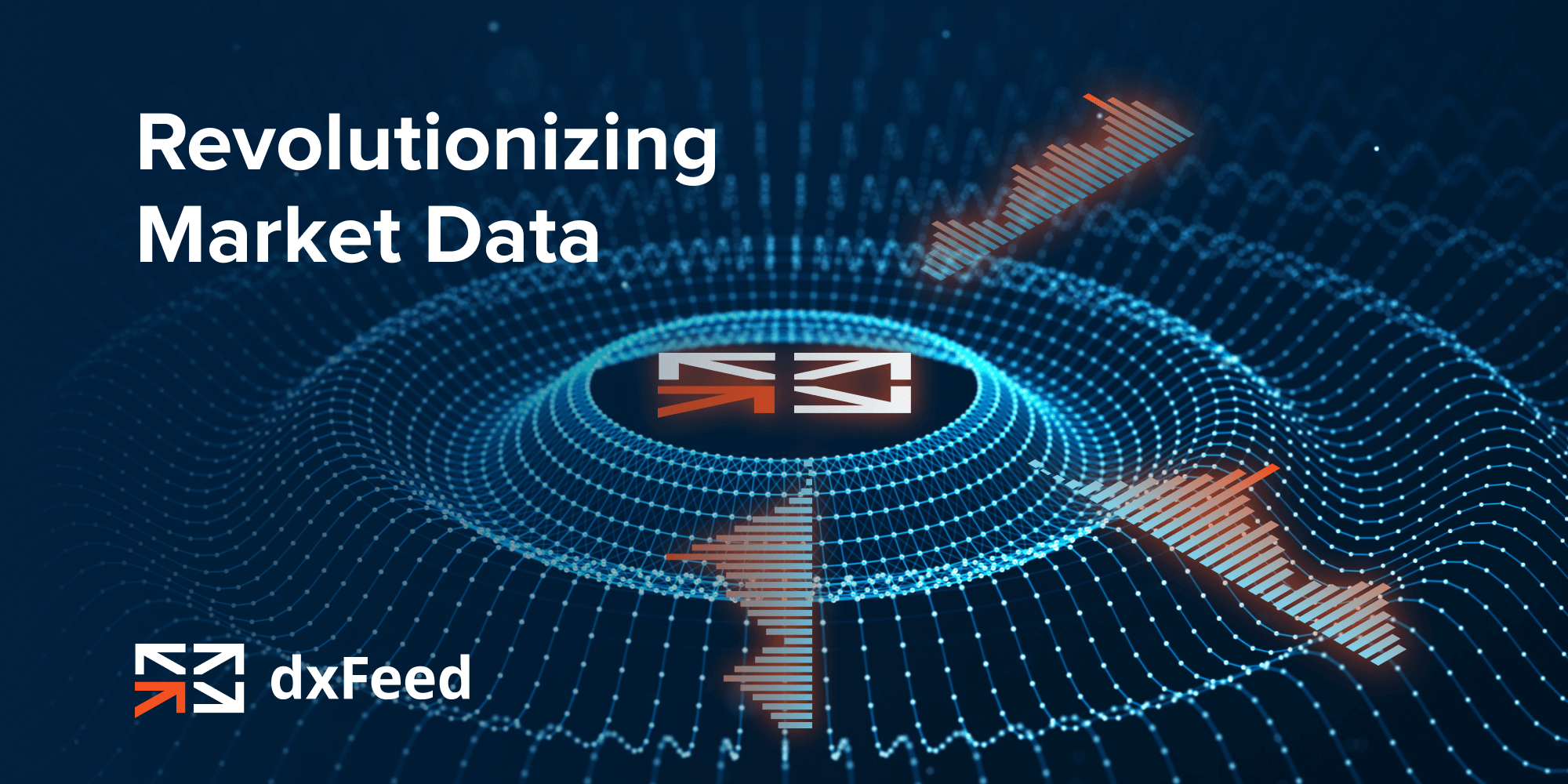
The advent of the Historical Data Lake (HDL) at dxFeed marks a significant leap forward in the realm of market data solutions. Just as a lake might appear calm on the surface, beneath the still waters of HDL lies a powerful and dynamic force capable of revolutionizing the way financial institutions, researchers, and data scientists approach historical market data. While lakes are traditionally serene, with HDL, it’s time to prepare to surf the waves of high-performance data access and analysis. Offering seamless, instant access to vast and complex datasets, HDL provides the tools and flexibility needed to tackle even the most demanding market data challenges—ushering in a new era of efficiency, scalability, and innovation.
HDL serves as a crucial tool for a variety of stakeholders, from asset managers and hedge funds to research institutions, trading firms, and clearing houses. It provides a centralized, high-performance repository for extensive historical market data that can be used for tasks like quantitative research, compliance, and managing risk. In addition to supporting data sales, HDL offers data snapshots that aid clearing firms and exchanges in reconciling transactions and managing risk. Its utility extends further by allowing backfilling of historical data for new platforms, bolstering market surveillance efforts, and enhancing client support by offering quick access to accurate historical records.
By making vast amounts of historical data readily available and easily accessible, dxFeed’s HDL opens up new revenue opportunities, facilitates market surveillance, and supports comprehensive reporting requirements, thus becoming an indispensable asset to the broader financial ecosystem. This article delves into why and how HDL has made life easier for index managers, provides insights into its technical underpinnings, and explores its broader impact on the market data ecosystem.
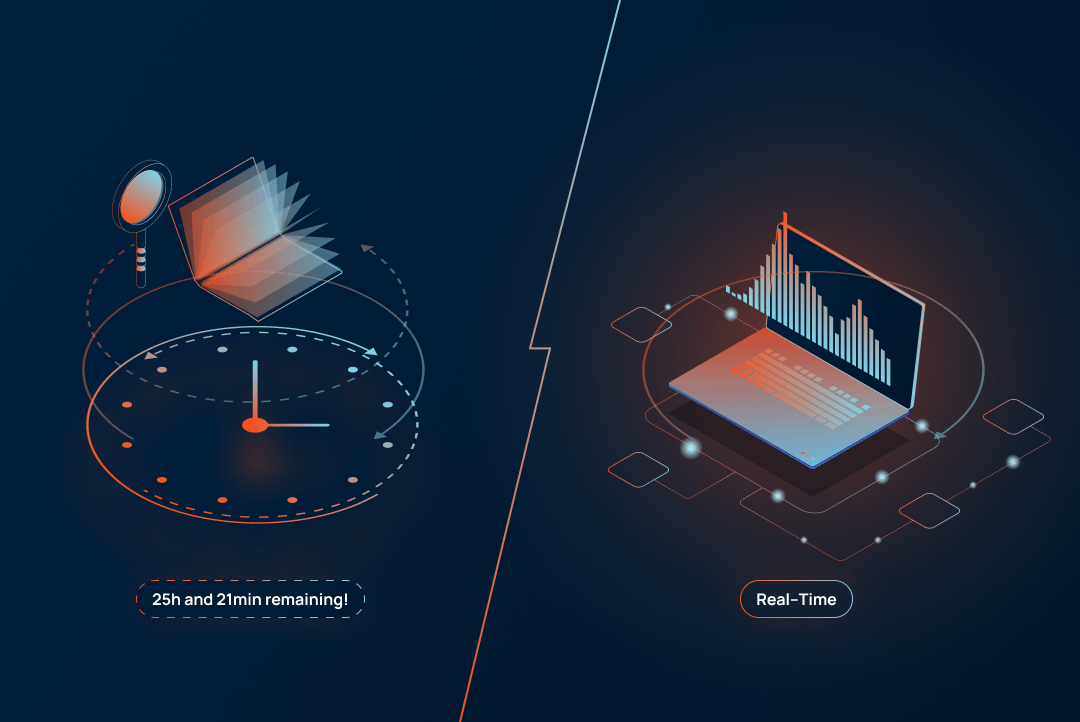
Transforming Index Management with HDL
Index Management is a domain where precision, reliability, and speed are critical. Traditionally, the backtesting of indices, models, and algorithms required significant time and resources, often hampered by a number of inefficiencies. One major challenge has been the limited accessibility to deep historical data, particularly when attempting to analyze events that occurred further back in time. As time passes, data becomes harder to retrieve, with archives often ‘frozen’—stored in formats that are cumbersome to access and process quickly. In many cases, extracting even a single data point from these archives could take hours or even days, delaying important research and decision-making. Moreover, such legacy systems often fail to provide the granularity needed to conduct deep dives into tick-by-tick or order-by-order data, forcing analysts to rely on aggregated datasets that lack critical details.
HDL, with its centralized storage and high-performance computing capabilities, has completely transformed this landscape. By eliminating the bottlenecks associated with traditional data retrieval, HDL enables users to access and analyze even the most granular historical data—regardless of how far back in time it goes—almost instantly. The ‘defrosting’ of data archives is no longer an issue; HDL keeps data readily accessible and easy to query, making it much more efficient to conduct thorough backtesting and stress testing. This streamlined approach not only saves time but also enhances the quality and accuracy of analysis, empowering index managers to make more informed decisions.
Additionally, a centralized index management platform built on HDL would enable more efficient backtesting, administration, and development of indices.
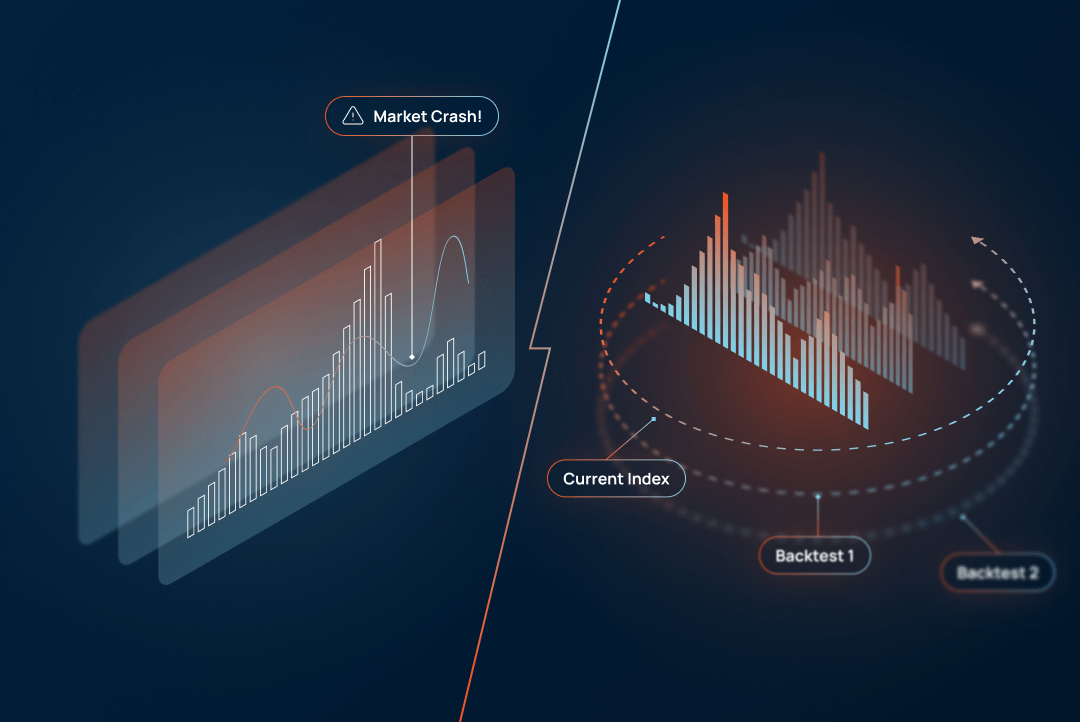
Enhanced Backtesting Capabilities
HDL allows for quicker and more reliable backtesting across longer historical time horizons and broader instrument universes. Imagine being able to test how your index would have performed not just last year, but 20 years ago—across different market conditions, economic cycles, and even financial crises. This is the power of HDL: it gives you the ability to ‘time travel’ through the markets, testing and refining your index against decades of historical data to ensure its robustness. This capability is crucial for constructing various versions of the same implementation, optimizing hyperparameters for key metrics such as performance, risk, and volatility, and ultimately selecting the best-performing models.
With HDL, it’s feasible to conduct extensive stress testing and run simulations over a wide range of scenarios, enabling a much more thorough evaluation of your end product. You can evaluate the robustness of your index by seeing how it withstands not just short-term fluctuations but long-term market dynamics through different volatility regimes.
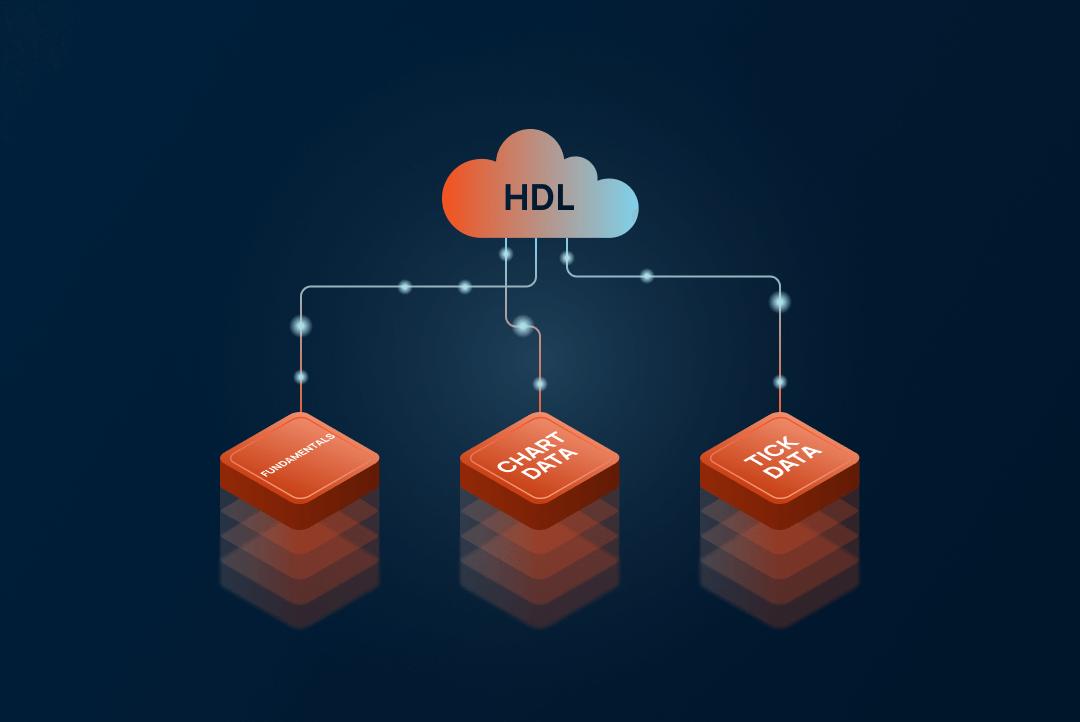
Unified Data Access and High-Performance Computing
One of the most significant advantages of HDL is its support for high-performance, distributed computing, making sophisticated data processing available on demand. With HDL, users can run complex models directly on raw tick-by-tick data, rather than relying on pre-aggregated data flows, resulting in far more granular and accurate analyses. This level of detail unlocks deeper insights that are particularly valuable for high-stakes scenarios like algorithmic trading and quantitative research.
What sets HDL apart is its ability to seamlessly handle Big Market Data. It brings together vast volumes of different data types—whether it’s tick-by-tick trading data, chart data, or fundamental data—in a single, unified platform. This combination allows researchers, analysts, and firms to perform comprehensive analyses, discovering insights or anomalies that only become evident when multiple datasets intersect. For instance, you can analyze price movements alongside order book activity and corporate fundamentals, all within the same environment, enabling more informed and strategic decisions.
Moreover, these high-performance computing resources and data access are available on request, meaning that firms and researchers of any scale can tap into the same powerful tools. Whether you’re testing intricate trading algorithms or conducting large-scale research, HDL provides the infrastructure to efficiently manage complex datasets, eliminating the need for costly in-house computing resources.
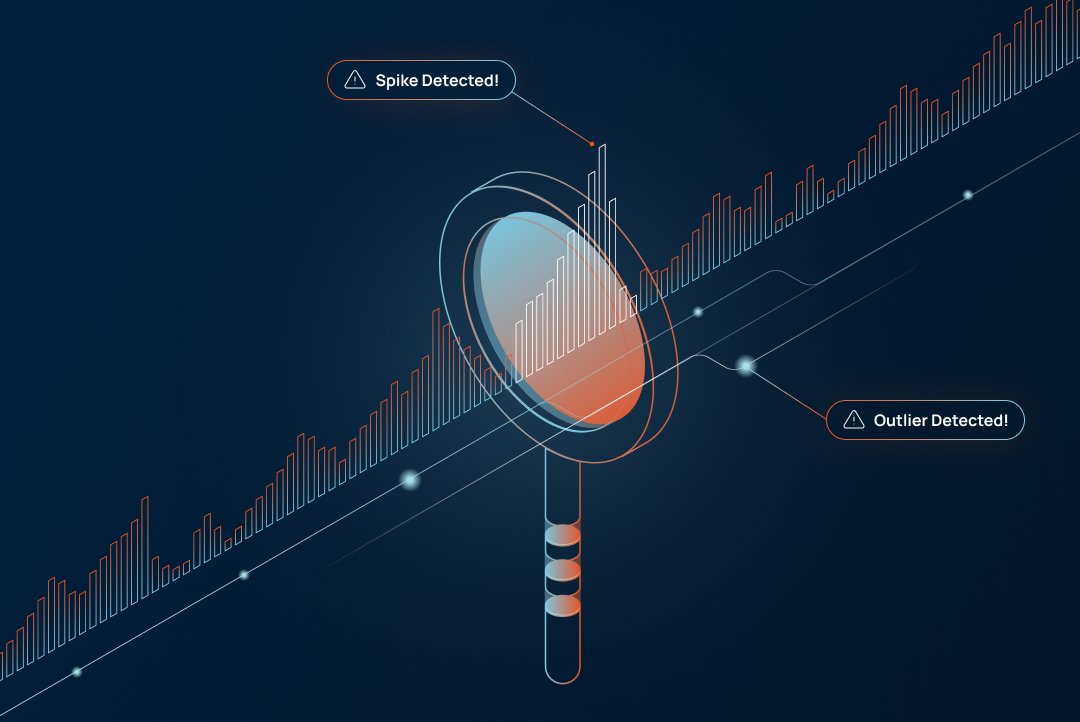
Improved Data Quality and Anomaly Detection
HDL also simplifies the process of running quality checks on data from various vendors. By identifying and addressing data problems or anomalies, dxFeed ensures that end customers receive accurate and reliable data. This proactive approach to data management reduces the risk of errors that could impact the performance of indices or trading algorithms.
In summary, HDL has led to significant cost reductions in running existing analyses, and enabled more complex computational tasks. These improvements translate into faster time-to-market for new indices and a more flexible and scalable environment for developing innovative multi-asset index methodologies.
The Revolutionary Approach of HDL
At its core, HDL is more than just a data storage solution; it’s a comprehensive platform that redefines how historical market data is managed and utilized. Let’s explore some key technical aspects that make HDL unique.
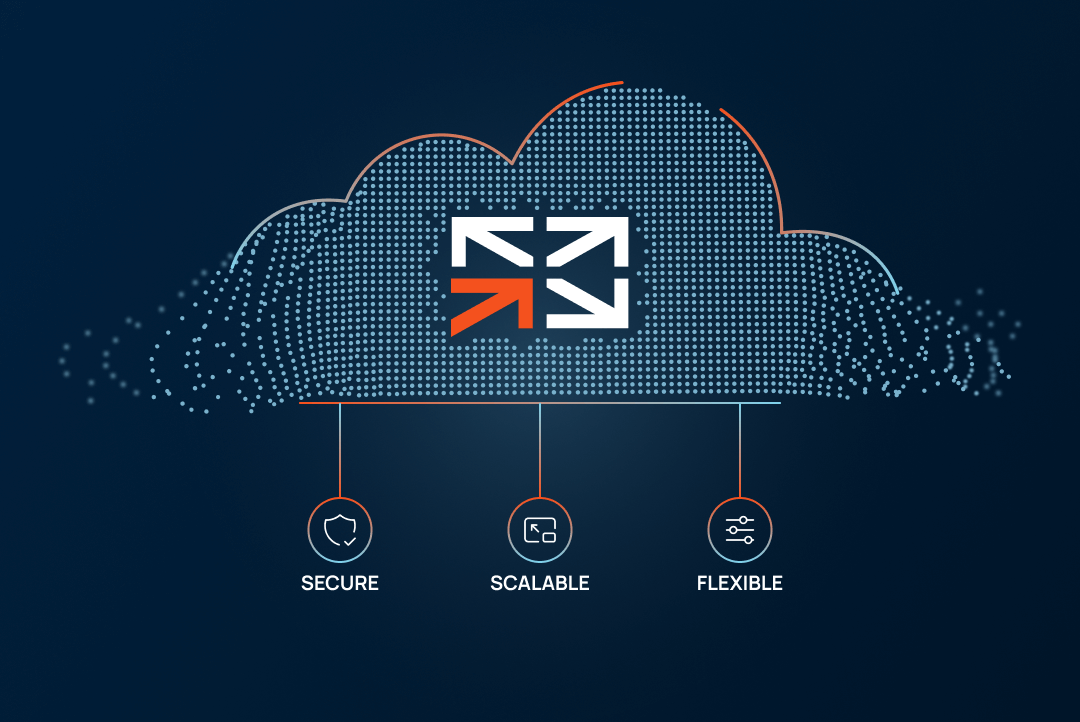
Robust Data Integrity and Scalability
HDL leverages most relevant data lake technology, which ensures data integrity through ACID transactions. This guarantees consistency even during concurrent read/write operations, making HDL a reliable and scalable platform capable of handling large volumes of data. Its ability to enforce and evolve schemas dynamically adds another layer of flexibility, accommodating changes in data structure without compromising stability.
Simplified Data Management
The transaction log within HDL tracks all data changes, simplifying audits and enabling easy rollbacks when necessary. This feature is particularly valuable for maintaining data accuracy and accountability, essential elements in a highly regulated environment like financial services.
Support for Batch and Streaming Workloads
HDL’s support for both batch processing and real-time analytics allows for a wide range of use cases, from historical data analysis to real-time market monitoring. This dual capability ensures that users can access and process data as needed, whether for immediate decision-making or long-term research.
Cost-Effective Storage and Data Accessibility
By reducing costs associated with data transformation and supporting long-term retention, HDL offers a cost-effective solution for historical analysis. Additionally, its compatibility with Apache Spark facilitates easy access and processing of complex data, making it an ideal tool for data scientists and financial analysts alike.
What makes HDL truly versatile is that its approach is not limited to dxFeed’s own data or market data alone. The platform is flexible enough to integrate data from any client, allowing firms to store, manage, and analyze their proprietary datasets alongside market data within the same high-performance environment. Moreover, HDL can be tailored to meet specific client needs, with the option to set up a separate platform dedicated entirely to an organization’s internal data management. This capability opens up new opportunities for clients looking for a scalable, high-performance solution to store and process large volumes of data efficiently, whether for financial markets or other industries
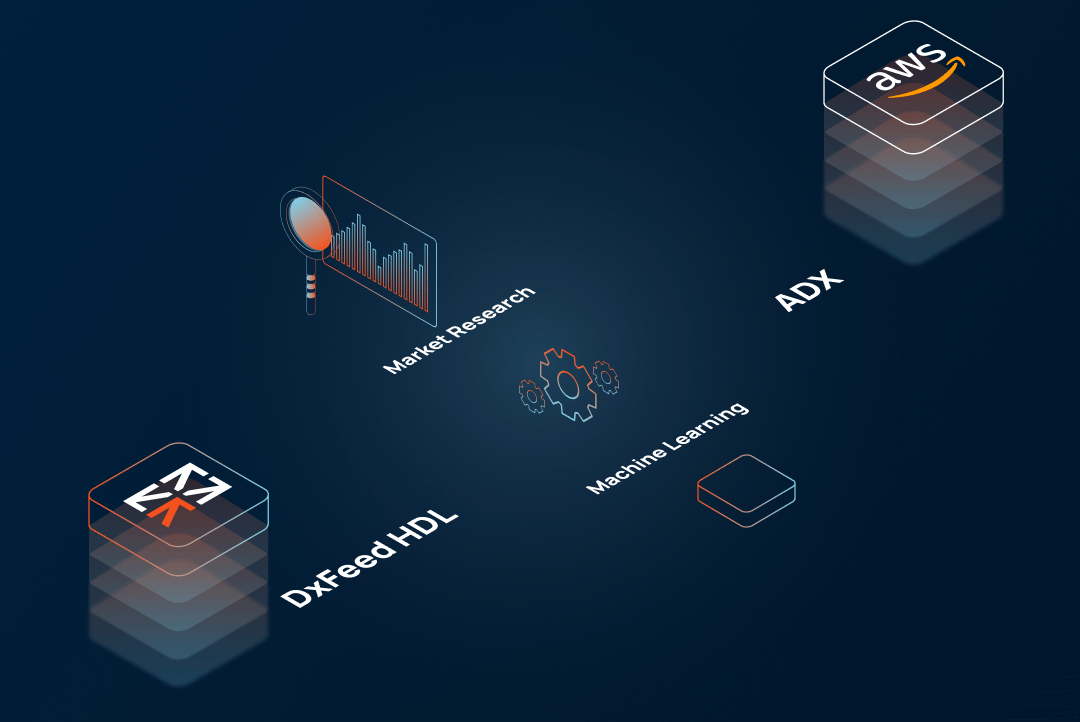
Integrating ADX for Broader Reach
One of the standout features of HDL is its integration with Amazon Data Exchange (ADX). This integration enables the retail sale of data through ADX, offering both static and dynamic data products. By making historical market data readily accessible through a trusted platform like ADX, dxFeed opens up new opportunities for customers to leverage this data for various applications, from machine learning model training to quantitative research.
In addition to accessibility, HDL offers a vast array of data types and outgoing formats compatible with industry standards, ensuring seamless integration with clients’ existing workflows. This broad data offering enables customers to solve their tasks more efficiently, with access to a wide range of financial instruments and historical datasets in formats they are already familiar with. Whether they need granular tick data, fundamental company information, or aggregated market insights, clients can access the exact type of data they need, all through a more convenient and cost-effective solution than traditional data providers.
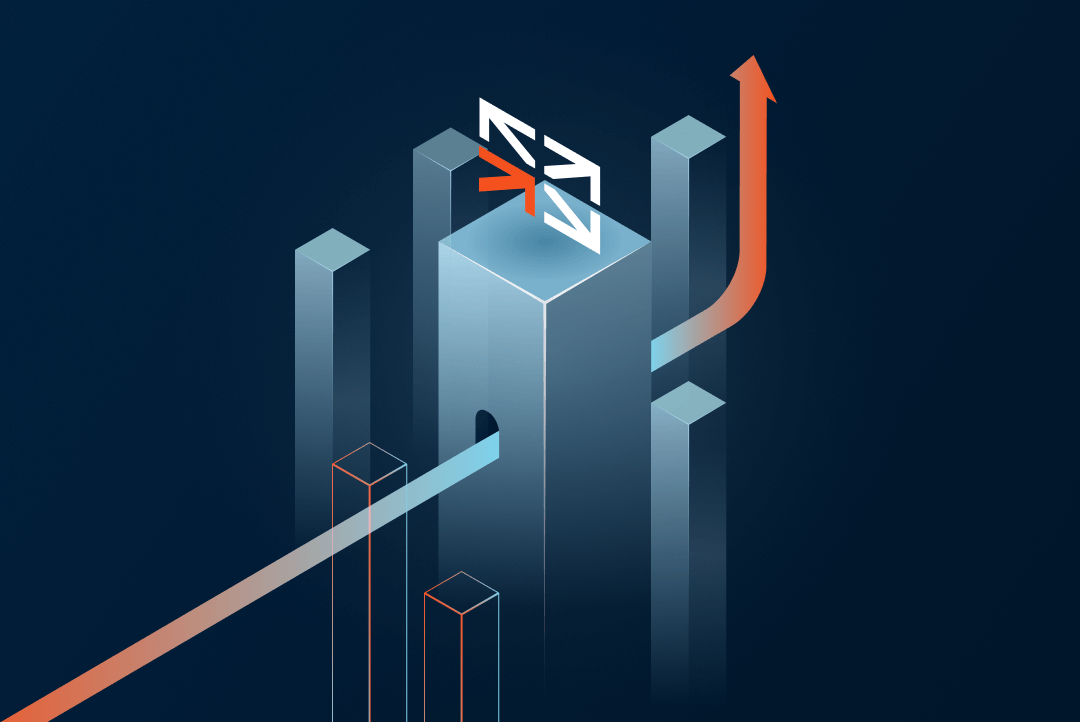
Future Development and Opportunities
Looking ahead, dxFeed has ambitious plans to expand the capabilities of HDL. These include supporting additional data sources, developing new data products, and enhancing tools for deep historical analysis. The vision is to create a generic data lake that streamlines data extraction, analysis, and management, ultimately serving as a platform for a wide range of market data applications.
AI/ML Model Training
With the vast amounts of historical data stored in HDL, there is immense potential to train machine learning and AI models that can predict market trends, identify trading opportunities, and optimize investment strategies.
Market Surveillance
HDL is also poised to play a crucial role in market surveillance, where it can help identify irregularities and provide alerts to regulators and market participants.
Conclusion
The Historical Data Lake (HDL) at dxFeed represents a groundbreaking shift in how historical market data is stored, managed, and utilized. By offering enhanced backtesting capabilities, robust data management, and seamless integration with platforms like ADX, HDL not only makes life easier for index managers or financial analysts, but also opens up new possibilities for innovation in the financial industry. As dxFeed continues to develop and expand HDL’s capabilities, it is set to become an indispensable tool for financial institutions, data scientists, and researchers alike, driving the next wave of advancements in market data solutions.