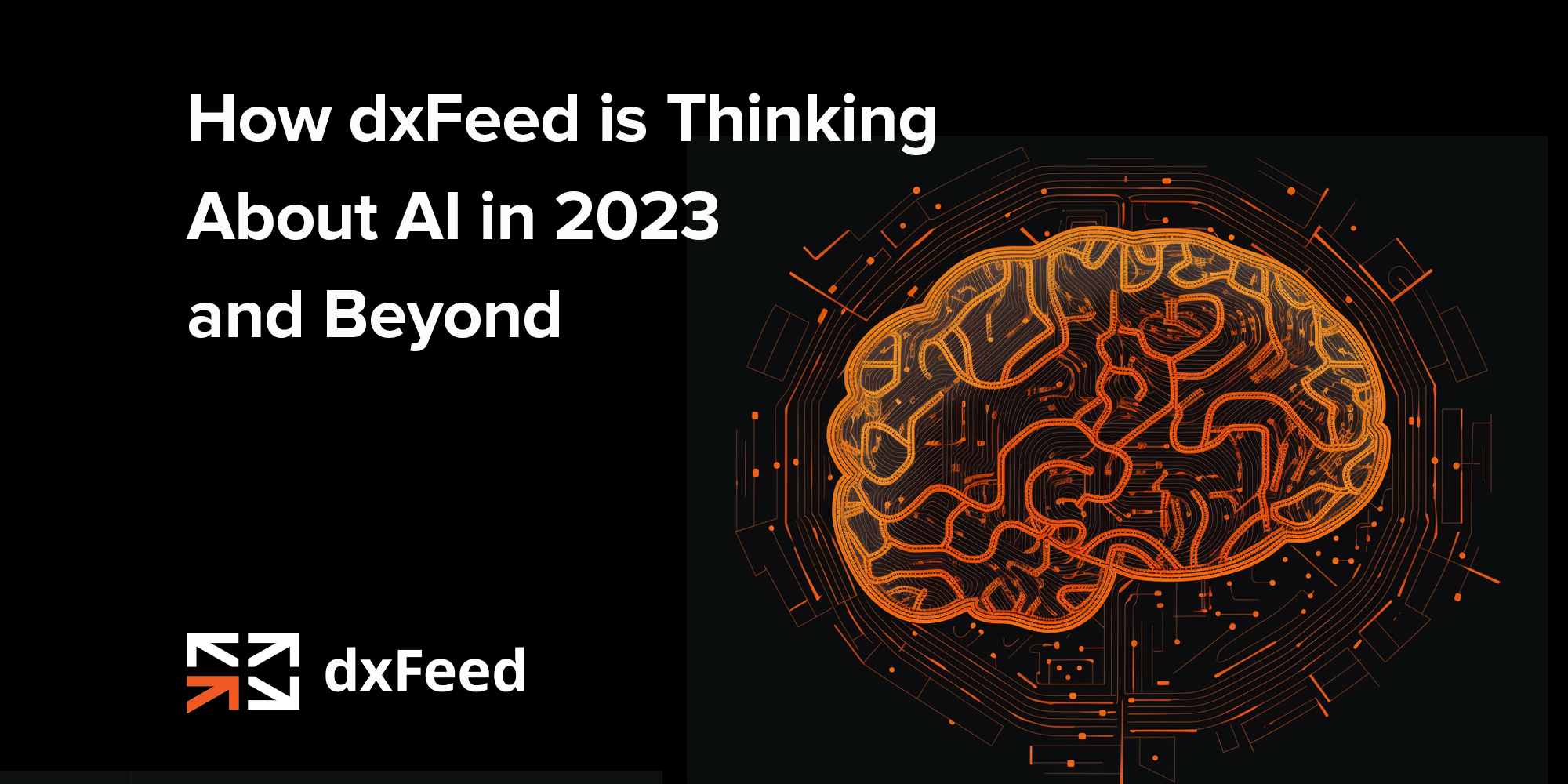
The large language model revolution begins
Artificial intelligence has been front and center this year, with several different large language models having undergone highly public rollouts. This has given users from all professions and walks of life an opportunity to test the technology for how it can offer efficiencies within their chosen domains.
OpenAI’s ChatGPT, which currently appears to be ahead of the competition in terms of its competence and mindshare, became the fastest growing consumer application in history when it reached 100 million users in January of this year, just two months after its launch.
The choice to make these systems publicly available for testing has also allowed the hype to spread like wildfire as users have tended to become some of the staunchest evangelists for the technology after experiencing it. The tens of millions of daily queries these systems receive are also an invaluable source of training data, helping their engineers iteratively improve upon them with a deluge of real-life examples to go on.
The lay of the land
The first inning of public and institutional experimentation with this technology has brought a number of important questions to the table that participants will have to navigate, going forward.
The first of which is the cost of queries, which are currently an order of magnitude more expensive than traditional keyword searches. If search is one of the ripest verticals for disruption by this technology, then the disparity between the cost of a Google search, for example, and a ChatGPT query will have to narrow.
A host of copyright issues are also naturally coming to the fore. The ways these models reappropriate existing web content in order to provide their responses raises new questions regarding ownership, licensing, and attribution, particularly when responses are being used for profit.
This naturally leads to the topic of proprietary data, which is to be one of the key drivers of AI models in the future, and is likely to be more important than hardware or even software in the long run. As these systems evolve they will converge in their capabilities, making the underlying datasets they have at their disposal the key differentiating factor between one system and another.
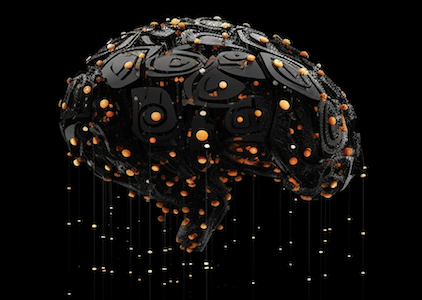
Proprietary data and domain specificity
This last point is precisely where companies such as dxFeed come in. As a vendor of premium market data and a creator of a variety of exclusive benchmarks across asset classes, dxFeed possesses a wealth of proprietary data that it can leverage to provide much more powerful responses within the domain of finance than a more general transformer model would be able to.
Those of you who’ve experimented with existing large language models are bound to have been tempted to ask for financial forecasts. If your question has even slightly skirted the topic of market predictions, you may be familiar with the “As an AI language model, I don’t have the ability to predict the future…” disclaimer that ChatGPT rapidly fires off in response to such questions.
The limits of these systems in this area run deeper than just their seeming unwillingness to field speculative questions. Their uncanny ability to appear instantly knowledgeable on a myriad of topics is what disadvantages them when it comes to achieving sufficient depth on any specific one. They do an amazing job at synopsizing the main talking points of any given subject, which to a lay person can seem astonishing, but anyone with a degree of domain expertise can almost instantly spot inaccuracies and equivocations.
Enter dxFeed AI
Our own platform, dxFeed AI, unburdened as it is from having to be all things to all people, has proven itself to be quite adept at stock market analysis. It enjoys privileged access to higher quality market data, combined with domain-specific knowledge on how to interpret it. This allows it to do a far better job in the more constrained environment of stock analysis than the large language models generating so much interest are currently able to.
In short, it picks a great stock, but it probably won’t fetch you the best recipe for crème brûlée, or be able to outline the main causes of WWI.
By analyzing technicals, fundamentals, news, and sentiment data, dxFeed AI is able to perform counter-intuitive comparisons, identifying patterns and correlations that may not be immediately apparent through a more traditional lens.
This is due to a network of associations that take historical price action, company metrics, news, and social sentiment into account, and combine these disparate data into knowledge graphs used for model training.
dxFeed AI has access to comprehensive stock market fundamental data and knows how to evaluate it. It can analyze vast quantities of company financial statements, current and historical, across an astonishing number of individual symbols, allowing it to make connections and identify trends that are simply beyond the capability of human analysts, however experienced they may be.
It also has access to complete symbol histories, allowing it to inform its longer-term fundamental outlook with a shorter-term technical view of price action.
The system is also equipped to perform market sentiment analysis. Using natural language processing, it’s able to monitor news and social media feeds, gauging real-time sentiment surrounding companies and use these insights to place fundamental and technical insights such as revenues, earnings-per-share, and oversold/overbought conditions into a broader context of investor bullishness or bearishness.
Achievements and roadmap
Thus far our team has succeeded in creating a data processing pipeline for converting reference, market data, news, and social network data into knowledge graphs for use in model training.
This has led to the development of a Machine Learning model capable of comparing two stocks and verbalizing the results in English, as well as a user-friendly web UI and HTTP server allowing third party API access to the system.
As part of dxFeed AI’s ongoing development, more pertinent market data is being made available to the system and integrated.
We’re also in the process of developing an embeddable user interface for the purpose of B2B integrations, but we also aim to launch a direct-to-consumer product for individual traders in the near future.
Conclusion
We believe that our dxFeed AI system demonstrates that high-quality proprietary data, in combination with domain-specific Machine Learning approaches, can provide a measurable benefit to stock market investors.
The ability of these systems to analyze vast amounts of data, observing trends and correlations at a resolution that eludes human analysts, is a game-changer in the realm of market analysis. Just as the new generation of large language models are currently proving to be game-changers in the fields of content creation and search, we believe that we are on the verge of creating a variety of AI market analysts/assistants that will revolutionize how traders develop their market outlooks in the future.